Media
The Definitive Guide to Understanding Incremental Return On Ad Spend (iROAS)
Written by Fred Askham ·
May 2021
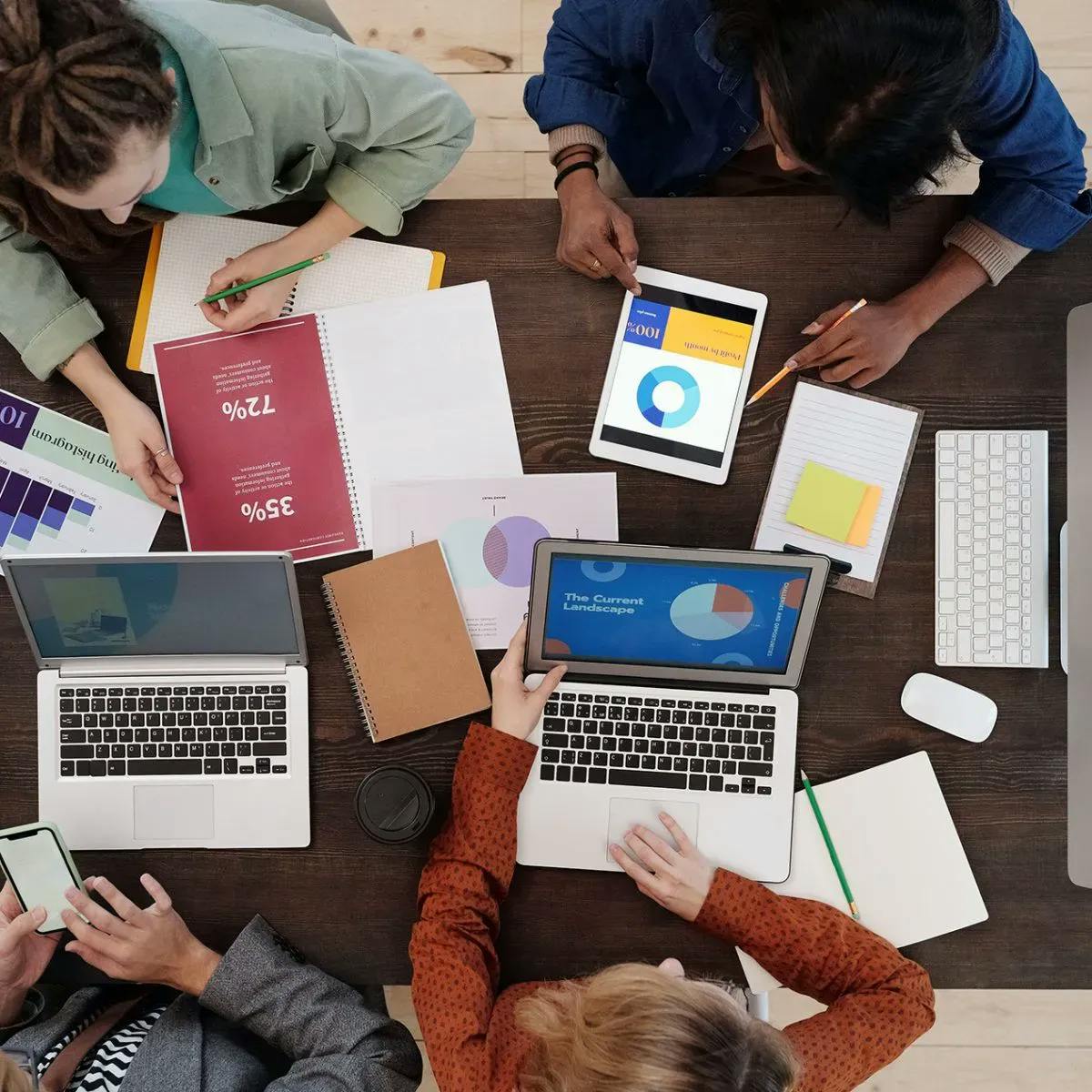
Incremental Return on Ad Spend, or iROAS for short, is one of the newer media performance indicators on the block. Naturally, we have received a plethora of questions from client brands and agency partners who want to know what iROAS is, how it expands our view of which media tactics are and are not performing, and what it brings to the table for forming a more macro understanding of a brand's success. If you are wondering the same thing then this article is likely for you!
What is Incremental Return on Ad Spend (iROAS)?
When it comes down to it, incremental return on ad spend is a reasonably simple metric. It is calculated in essentially the same way as Return on Ad Spend, making it familiar for those who have experience with that metric. The formula is as follows:
Incremental Revenue/Spend = iROAS
The nuance of this metric rests in the very first word in the formula: incremental. To understand iROAS and its implications, we must first understand what incremental revenue is.
Incremental revenue is the additional amount of revenue that a brand only received due to its marketing efforts. If you are a brand manager, think about what sales would look like if you simply shut off all of your marketing efforts. Now think about what they are with your marketing efforts on. The difference in sales between those two scenarios is the incremental revenue generated by marketing.
In essence, iROAS is a measure of any given marketing effort's impact on revenue. A high iROAS figure indicates that a marketing tactic is effectively driving growth. On the other hand, a low iROAS figure indicates that a tactic may be targeting people who aren't willing to buy a brand's product—or that they would have bought it regardless of marketing (more on how to suss out that nuance later!)
In essence, iROAS is a measure of any given marketing effort's impact on revenue.
This concept makes intuitive sense to most, so the problem is determining how to accurately measure the ongoing impact of a brand's marketing efforts on an incremental basis. Different strategies for measuring incrementality can affect both the overall accuracy as well as the granularity of the insights that can be provided through your digital marketing attribution platform.
Measuring Incrementality - Three Systems
When it comes to measuring incrementality, marketers can leverage three distinct platforms or methodologies to gain quantitative data.
The first and most commonly known method is MMM (marketing mix modeling), which assesses incrementality through an econometric modeling approach. MMM has been widely adopted by large CPG, retail, and pharma brands over the last 20 years. It is most effective when two criteria are met:
- The marketer is analyzing a very large paid marketing budget
- The vast majority of relevant marketing efforts are non-trackable
Marketing efforts are generally non-trackable due to how they are delivered to consumers or because product sales are not directly trackable. The most concrete way to think of this is if marketing is largely non-digital, or sales happen primarily through distributors or non-owned brick and mortar locations, then MMM is likely a good option. The drawbacks are that the incrementality results are relatively high level (think primarily inventory type-level - e.g. display, or network tv) and the results take a relatively long time to produce. Updates of the model are typically quarterly, making on the fly optimizations in digital advertising based on these systems near impossible.
The second option is a method called Public Service Announcement (PSA) testing. Strangely, the testing has nothing to do with the efficacy of the PSAs themselves, but rather uses PSAs to form test groups for more granular digital marketing incrementality tests. Think of the system this way: one part of your audience only receives PSAs, while another part receives branded marketing. From there, you simply measure the difference in conversion rate between the two groups.
The PSA group represents the audience's baseline propensity to buy from your brand, so any gap in conversions between the two groups represents the incremental lift from your marketing efforts. This system has several benefits. First, it allows marketers to get a more granular view of the effects of specific marketing tactics. That benefit is often offset by two major drawbacks, the first of which is that PSA testing costs a substantial amount of money to be spent on buying inventory for the PSAs. The second is that it requires part of your audience to be held in a control group, hampering your ability to generate outreach and putting a serious constraint on the speed at which marketing changes can be made. That is to say, there is a substantial amount of work involved in adding additional exclusions to all the paid marketing lines to ensure that the test results are not skewed by overlapping media buys.
The final method, causal attribution, is the most recent development in the space. It has primarily been driven by an increase in the measurability of marketing and marketing inventory over the past 3-5 years. At their most basic level, causal attribution systems measure incrementality in much the same way as PSA testing. The key difference is that they leverage a convenient statistics method known as the Rubin Causal Model to eliminate many of the drawbacks associated with traditional PSA testing.
While that may sound highly complex, it is actually quite simple. Think of it this way: instead of deliberately creating control groups, these models form your control groups from the data that your marketing campaigns are already creating. There are several ways that this can be done. One method involves looking at the conversion rate of consumers where an ad was purchased on the exchange vs. the conversion rate of consumers who were eligible for an ad to be purchased but one wasn't (ghost ads I have heard this method called in the past). Another would be to look at the conversion rates for consumers who were served viewable media vs. those who were never shown a viewable ad. Regardless of the exact method of separation between the groups, the point is that these platforms allow you to measure both baseline consumer behavior and consumer behavior after exposure to branded marketing (and thus incrementality) at a granular level, and they do so without the need for expensivee PSA ad placements or the restrictions of a control group. These factors have made the causal attribution methodology the favored approach for digital-first marketers and eTail focused brand managers.
Regardless of the system you use to measure incremental revenue, the uses of incremental ROAS metrics are largely the same.
How iROAS Eliminates a Key Vector of Measurement Bias
If you are like most people, you read that headline and immediately thought I hated statistics in college, TL;DR. But hold up for just one second because this concept is critical if you want to be successful and we have done our best to make it suck less.
So here it is straight.
Let's say you are optimizing your media to plain ROAS. What exactly does that mean? Well it means that you are spending your marketing budget more heavily on media tactics that are showing more ad-touched revenues and more ad-touched revenues per unit of advertising spend. To which you are now thinking well great, that is the point of my job, drive the most sales possible with marketing. What's the issue? To which we say measurement bias.
What is measurement bias? In short, we introduce measurement bias when the way that we measure something systematically skews our understanding of it. Case in point, buying non-viewable inventory against audiences who are already regularly purchasing your products will show a large plain ROAS metric (non-viewable inventory is cheap, and non-viewability of an ad doesn't restrict tracking from being activated and view-through conversions being attributed to those lines). The question then becomes, does that make buying non-viewable inventory against loyalist audiences an effective media strategy? Of course not! Not showing someone an ad is certainly never an effective use of marketing budget. But if you were optimizing towards ROAS the suggestion would be to allocate additional budget to these lines. The difference between the common sense optimization and the optimization recommendation based on ROAS is the result of that measurement bias. The way we are measuring ROAS in the example doesn't properly reflect what we know we want to achieve. While this is an extreme example, it does highlight the concept of measurement bias and how it can be detrimental. What is important to understand, is that this same measurement bias exists throughout the entirety of your digital marketing measurement to various degrees and is actively providing a drag on the quality of your optimizations if you are optimizing with plain ROAS metrics.
The implications? The growth you are driving with marketing is slower than it could be if you are optimizing to plain ROAS metrics.
iROAS, on the other hand, accounts for the baseline consumer propensity to buy your product, which is one of the most common sources of measurement bias in digital marketing. In our previous example of buying non-viewable inventory against loyalists, a good incrementality measurement solution would show a $0 iROAS metric (the worst possible) against that buy. Not only does that align the iROAS KPI more closely with what we know to be intuitively true in this example, it also means that there is significantly less error in how marketing budgets are allocated, and that leads to better long-term growth.
iROAS in Complex Environments
All this has been great so far, and it makes sense in simple examples. But what happens when we know that our consumer journey is complex, involves a large number of marketing and organic touchpoints, and our campaigns have an omnichannel bent? Basically, what happens in reality?
The answer is that multi-touch attribution (MTA) principles should come into play. What I mean is that incrementality and iROAS should be looked at in frameworks that are more complex than simple all-or-nothing attribution models. This will help us get the most out of our marketing efforts and continue to reduce our measurement biases.
As a framework for that discussion, it is important that we lay out the two types of multi-touch attribution models and discuss the methodologies of each and how that affects our results.
The first common MTA method is what is known as** rules-based distribution**. It is common because it is simple and easy to implement. If consumer A received 4 ads, each ad gets 25% (or 1/4) credit. These rules can get as complex and arbitrary as one might desire. Three times extra credit to the third impression in the journey only if it happened on a Tuesday during a waning moon! Joking aside, complex sets of rules often do the very opposite of what they are intended to, adding back in measurement biases instead of removing it.
The second method for MTA is what is known as data-driven distribution. This is what you will get from most MTA service providers like Nielsen or Google. Basically, the idea is to let the customer and how they act determine what media and marketing efforts should get credit. Nielsen which was formerly ViQ uses a latency-based approach to weigh the credit to impressions. Google, which was formerly Adometry, uses what is best described as a Markov Chain approach. We prefer Google's approach, but regardless of the specifics, the concept of letting the quantitative information on consumer behavior decide which marketing tactics get credit is the important value driver.
This concept applies to iROAS because it is possible to implement both incrementality measurements and MTA into a single platform. The result is the fractionally attributed iROAS for each media line. That allows us to understand both how incremental our audience targets and marketing efforts are, as well as how well each of our specific tactics or channels is reaching and influencing that audience.
How to Optimize for iROAS
Now that we have discussed the intricacies of what iROAS is and the mechanisms that allow it to have a real impact on the marketing efforts of brands using it, it is important to discuss how one actually does use iROAS once they have it.
The bottom line is that this can be broken down into three major categories:
-
Using iROAS to make granular platform level adjustments
-
Using iROAS to make budget flighting decisions
-
Using iROAS to find additional strategic opportunities
The switch is generally very simple when it comes to using iROAS to make granular platform-level adjustments. The standard dominant auction strategies should be leveraged with iROAS (or iRevenue) in place of ROAS (or ad-touched revenue). For reference, in 2nd price auction environments, that strategy is to bid one's observed value to gain maximum surplus. In 1st price auctions, the dominant strategy is dynamic price shading.
As it relates to budget flighting, the optimal result is to flight spend such that iROAS is flat across all periods. This requires brands to heavy up advertising costs during seasonal periods of high iROAS values (the diminishing returns on higher ad spend pull the seasonally higher results down) and to cut down advertising spends during seasonally down periods focusing on just the most incremental groups. Leveling out iROAS to account for seasonality provides the highest possible return for the brand and effectively drives growth. Gathering the proper ad server logs now will ensure that even if you don't implement a causal attribution platform in the near future, your historical data will be able to be processed so that you have a basis for planning against these targets.
Finally, iROAS can also be used in a comparative framework to generate deeper and more complex marketing strategies. For example, on lines where we see high ROAS metrics and low iROAS metrics, we know that there is a high baseline propensity to buy your products—the high ROAS implies that sales are happening often, while the low iROAS indicates that something other than marketing is driving the results. Perhaps these targets would be a good place to initiate a refer-a-friend campaign since they obviously carry brand loyalty, or as the base for a subscription-driven model for your products since they have a consistent need. Alternately, concurrent low ROAS and high iROAS metrics imply that your audience is generally skeptical of buying your product. On the other hand, the few who are convinced are new to the brand. This would be a good place to shift the focus of your messaging to different product or service value props to see if you can address the skepticism and drive improved product trial rates.
Final Thoughts
iROAS is an incredibly powerful metric for etail and eCommerce brands to leverage in their ad campaigns if they want to continue to scale their return on investment from marketing. This has become especially true during the pandemic as etail and eCommerce have become consumer habits and shifted the way that brands will sell to consumers for the foreseeable future.
We understand that the complexity of iROAS the systems that make it accessible can be a daunting labyrinth to navigate, and we sincerely hope that this guide has at least helped you on that journey. We have seen firsthand how impactful these systems are for our partners and clients' businesses and would love to see you have that same success whether that is with us or not! That said, if there is any other way that we can be of assistance to you on this front, please do not hesitate to reach out and ask!
Share this post